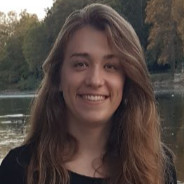
Mechanical properties prediction for oxide glasses assisted by machine learning
Morgane Rondet postdoctoral fellow at Mines Paris, PSL University will present her research work at the next Magma/Geodynamics seminar.
Room E001
Abstract
Morgane Rondet (1), Franck Pigeonneau (1), Elie Hachem (1), Olivier Delataulade (2)
(1) Mines Paris, PSL University, Centre for material forming (CEMEF), UMR 7635 CNRS, 06904 Sophia Antipolis, France
(2) L’Oréal, 9 rue Pierre Dreyfus 92110 Clichy, France
Glass has been around for thousands of years and is used in many areas including housing, packaging, automobiles, aeronautics, telecommunications and even cosmetics. Although it requires a lot of energy, this durable material has environmentally friendly properties. It’s chemically neutral, impermeable to gases and can be recycled indefinitely without changing its composition or properties. Unlike glass, plastic is an inexpensive product with many qualities, but it remains extremely polluting. Plastic is a material used in many fields.For ecological reasons, we want to replace plastic by glass packaging, while maintaining the same characteristics (weight, mechanical resistance). In addition, reducing the weight of glass packaging leads to a reduction in raw materials, but also a significant environmental impact. Lightweight glass is one of the challenges of our time to reduce the carbon footprint of the industry. Despite the intensive use of oxide glasses in industry, the composition-property behavior of these materials is still poorly understood, in particular for lightweight impact-resistant glasses.
New glasses require an understanding of how the composition of a glass determines its properties such as density, toughness, Vickers hardness or glass transition temperature. The creation of glasses for specific applications is still hampered by the lack ofcomposition-property models. Two methods have used to achievethis project. The first is the use an artificial intelligence tool with neural networks model(Ravinder et al.2020)and the second is the improvement of an existing physical model (Makishima and Mackenzie 1973). In the first step, from a database containing a range of oxides with different physical properties,several prediction modelshave been testfor density, Young’s modulus, glass transition temperature and Vickers hardness.
The four models developed show a non-linear composition-property relationship and excellent predictability. For the second method, we developed a factor based on the Makishima and Mackenzie (1973) model that can be adapted to any composition to calculate the Young’s modulus. The final objective is to invert the models and predict the composition of the glass from the physical properties, thus enabling the design of ultralight glass.
References:
Makishi Makishima, A., and J. D. Mackenzie. « Direct Calculation of Young’s Modulus of Glass ». Journal of Non-Crystalline Solids12, no 1 (1973): 35-45. https://doi.org/10.1016/0022-3093(73)90053-7.
Ravinder, R., Karthikeya H. Sridhara, Suresh Bishnoi, Hargun Singh Grover, Mathieu Bauchy, Jayadeva, Hariprasad Kodamana, and N. M. Anoop Krishnan. « Deep Learning Aided Rational Design of Oxide Glasses ». Materials Horizons7, no 7 (2020): 1819-27. https://doi.org/10.1039/D0MH00162G.